Mobility data management and its potential to generate value
Data is a promising game-changer for future mobility. Effective data sharing between cities, public transport operators and private mobility service providers has the potential to boost better mobility management while enhancing the competitiveness of private stakeholders. This article provides a description of the different barriers that mobility stakeholders, both public and private, must overcome in order to unlock the value that data can provide to improve the business models of mobility operators and support informed urban mobility planning. In addition, the proliferation of micromobility services reshaping urban mobility generates a need for policymakers to understand these new trends by requesting data of mobility operators while ensuring fair data-informed mobility policies. This article also goes into depth on such intangible aspects as trust, which plays a key role in unlocking value from sharing data. Why are operators reluctant to share their data? How can users’ privacy be protected and operators’ competitiveness preserved by anonymising data? The MDS and CDS-M initiatives propose ways to govern data sharing from shared service providers to cities. A consensus option is that all parties trust a third party that handles data. An analysis of pros and cons is provided, including real-world examples, highlighting the fact that there is no optimal option for all possible scenarios, because this depends on the level of risk and intervention that the stakeholders involved are willing to take. Data also plays a key role in enabling MaaS (Mobility as a Service), as increasing the availability of data is a precondition to achieving superior integration levels (from one to four): many cities already have access to mobility datasets from private mobility operators as a prerequisite for receiving a licence to operate in cities (Level 1). Level 2 uses available data to develop evidence-based decisions aimed at creating more effective mobility policies, but only a few cities have reached this stage through pilot projects. Finally, MaaS Levels 3 and 4 will add pricing strategies with the ability to influence mobility users’ behaviour and mobility management to promote societal goals through access to real-time data from various mobility services. The use of Software as a Service platforms such as the novel Rideal will play a key role in designing incentives programmes to nudge behavioural change towards more sustainable mobility.
Data is ubiquitous. We all unwittingly generate vast amounts of data when moving from A to B, whether by car, bike, walking, public transport, or other not-so-new mobility services. This mobility data has huge potential, which remains mostly untapped. Data empowers cities to make informed decisions in terms of mobility planning and management. For this reason, data sharing between cities and mobility providers –incumbents and new entrants– is of strategic importance. Data protection and privacy are, nevertheless, key issues in this context. Dependent on mutual trust, various models for mobility data sharing are feasible in the collaboration between cities and mobility providers. There is no clear best practice, as each case must be assessed individually. Only with the contribution of data provided by as many of the players involved as possible, Mobility as a Service (MaaS) at its highest levels of integration will succeed. MaaS aims to maximise public benefit, strengthen public transport, and meaningfully integrate private mobility services. Mobility providers and cities are dependent on each other for long-term, sustainable success that also affords social and environmental benefits, where cooperation and incentive schemes will be key to success. “Data is the new oil” is a trite mantra for the modern times. While huge value can be unlocked by ensuring access to curated data turned into actionable insights that mobility managers can leverage; do not forget the old but prevailing rule: rubbish in = rubbish out. Scattered, low-quality mobility datasets offered under not-so-open data portals are no oil to move anything, particularly if one would associate the idea of oil with some sort of monetary value. Moreover, in times when decarbonisation is top of mobility leaders’ agenda, data as oil is a deprecated metaphor. Oil is oldish. Words matter.
Getting around the city using new mobility services is becoming commonplace. With just a few taps on an app, users can today gain access to a wealth of ready-to-use vehicles just around the corner. Convenient. Swift. Fun. As users locate, book, pay for, and ride in shared vehicles, they produce masses of data. The same happens when they use public transport. Indeed, public transport authorities, operators, and cities worldwide have become open data pioneers by widely adopting de facto data standards and leveraging them in order to implement data-driven mobility strategies. Unfortunately, though, mobility data is still too often kept in silos, hindering its potential for gleaning deeper, actionable insight. New (and less new) private mobility operators are generally more reluctant to open their data, if not forced to or if the right incentives are not available (such as mutually beneficial Public-Private Partnership models towards the realisation of MaaS). For cities, access to data from mobility operators providing services in the public right of way is a priceless tool for enabling more informed mobility planning and management. For mobility operators, it can provide valuable information for enhancing their competitiveness and improving the efficiency of their operations.
Several hurdles must be overcome in order to unlock the (huge) expected value of mobility data: the lack of trust between the various players in the highly competitive urban mobility market, the need for standards enabling interoperability, and a level playing field setting the legal, regulatory, and technical conditions for effective, secure, and fair data sharing.
New mobility services continue to evolve and change the urban mobility landscape. This is especially true for micromobility. Micromobility refers to a range of transportation modes for short-distance travel using light vehicles typically operated by one person (e.g., bicycles and scooters). Today, these are often electric vehicles that are part of a self-service system and are rented by people for short-term use within a city. Given this, it is critical, and strategic, to leverage new mobility data to evaluate and understand how new mobility services are reshaping our communities in everything from equity, inclusiveness, road safety, and the environment (in other words, sustainable mobility).
Because cities did not act quickly enough in setting data sharing frameworks when ride-hailing companies burst onto the mobility market scene a decade ago, and with new shared mobility services still burgeoning (or in their infancy, if we are talking about micromobility), cities now have the unmissable opportunity to avoid repeating the same mistakes and so require the data they need to better inform their decision-making as regards mobility planning and management.
To do so, cities will need to set clear, indisputable data sharing requirements to mobility operators that identify the information they are seeking to acquire, how it will be collected, processed, shared, and stored, and the quality, accuracy, format (that is, standards), and frequency of the requested data, as well as determining clear privacy guidelines to protect both end users, but also the legitimate concerns from mobility operators regarding commercially sensitive data (Figure 1).
It is worth underlining the fact that the definition of personally identifiable information (PII) is rapidly changing and varies considerably between jurisdictions, so it will be important for cities to be clear about what they are to consider PII and how best to manage and protect this data, where GDPR has laid out a specific set of regulations dealing with PII.
Besides separate data sharing agreements with mobility providers, there are other innovative and promising ways cities can obtain coveted data. The recently started Cleanergy 4 Micromobility project, coordinated by Factual and co-funded by the EIT Urban Mobility, addresses this very specific aspect. The goal of the project is to develop an e-scooter docking station. These are designed to address the main problems of e-scooters and ensure orderly, safe, and sustainable micromobility growth. The docking stations will make e-scooter parking more organised and secure. They will also incorporate storage space for helmet provision and solar power generation so that e-scooters can be charged with green energy directly on-site. But the most crucial and game-changing aspect is data management. This is because the docks in which the shared e-scooters must be parked will belong to the city council. Hence, this model will lead to valuable information about the usage of shared e-scooters, as the city council will automatically have access to all the mobility data generated by each e-scooter that takes a trip from any dock to another.
Figure 1. A 4-step framework for effective mobility data sharing
Source: own elaboration from UC Davis
In mobility, one size does not fit all. Any framework for mobility data sharing implies that mobility operators and public authorities must cooperate and establish some level of trust with each other, where trust is linked to transparency in principles of purpose, use and data minimisation. We basically differentiate between three schemes, and it is ultimately up to the cities to define which model suits them best.
Mobility operators can provide the requested data to the city’s governing agency directly, and this can be done basically in two radically different ways, carrying different implications:
When operators do not trust public authorities or are reluctant to share their data because they feel that their users’ privacy or their competitive advantage might be compromised, they first “cook” data by aggregating it before providing a subset of data to public authorities, which might use it for auditing purposes. This is the case, for example, of Uber Movement, where the company releases datasets to the public in support of urban and transportation planning. To prevent user privacy issues, Uber aggregates car GPS traces into small areas and releases free data products that indicate the average travel times of Uber cars between them. Although this is certainly somewhat useful, some might consider it a PR strategy; some researchers have even pointed out the extent to which such data is actually helpful at all in supporting or informing any mobility strategy. Overall, it is quite evident that Uber and other mobility operators are generally rather reluctant to share raw data with public authorities, which is the next scheme we analyse, where…
Cities do not trust operators, so they mandate that operators send all the raw data they generate while operating their services.
A remarkable initiative designed to govern data sharing from shared micromobility service providers to cities is the “Mobility Data Specification” (MDS), “a digital tool that helps cities to better manage transportation in the public right of way. MDS standardises communication and data-sharing between cities and private (micro)mobility providers, such as e-scooter and bike share companies. This allows cities to share and validate policy digitally, enabling vehicle management and better outcomes for residents. Plus, it provides mobility service providers with a framework they can re-use in new markets, allowing for seamless collaboration that saves time and money” (OMF 2022).
The MDS has garnered a lot of attention from cities and the micromobility industry since it was introduced by the Los Angeles Department of Transportation (LADOT) in September 2018. It builds on the GBFS standard (General Bikeshare Feed Specification) by expanding on what additional data cities could require from mobility operators. Unlike the GBFS, in addition to the status of available vehicles, the MDS also specifies how information should be shared about vehicles that are unavailable due to redistribution, maintenance, or low battery through vehicle event status changes. The MDS has also introduced the concept of sharing data for trips, including starts, ends, and entire “breadcrumb” trip trajectories. While the MDS was initially designed for dockless bikes and scooters, and currently only specifies how data from these services should be shared, many cities hope to expand these data sharing standards to other services, such as ridesharing.
The MDS is not without controversy: concerns have been early raised by major players in the market, such as Uber, which have sued LADOT over what the company considers to be overly stringent data-sharing requirements. This is just one reason why the MDS is being examined by some EU countries to assess compliance with the GDPR, and the TOMP working group is working on the alternative City Data Standard for Mobility (CDS-M) API. The TOMP-WG (Transport Operator, MaaS Provider – Working Group) is “a collaborative initiative to create a standardized language for the technical communication between Transport Operators and MaaS Providers within the MaaS ecosystem by means of an API” (TOMP-WG 2022).
All parties may trust a third party to handle all data. This party may be an academic institution, a not-for-profit organisation, a public authority, or a data analysis platform, such as Fluctuo, Vianova, Populus, or Remix, to name but a few. This is usually a cost- and time-effective option for cities without the required data science expertise, but it could eventually lead to some sort of vendor lock-in. The much talked-about recent acquisition of Remix by Via, a Demand-Responsive Transport (DRT) service provider, for $100 million is just one example about how valuable data is for transportation planning. Mobility operators (like Via) can then leverage such data to provide evidence to cities regarding the convenience of incorporating their services. Also, notably, Factual joined forces with several other stakeholders under the MultiDEPART project, co-funded by the EIT Urban Mobility, to develop a dashboard and tools for cities to plan, manage, and monitor vendor-agnostic DRT solutions.
So, of the three described approaches to mobility data sharing, the decision of which to pursue ultimately comes down to how much risk, and how much active intervention, in terms of data processing and analysis, cities are prepared to take. The price tag (to be) associated with mobility data remains one of the most complex issues to address (Figure 2).
Figure 2. Pros and cons of the three approaches to mobility data sharing
Source: own elaboration based on the International Transport Forum and Populus
Acknowledging that an effective trust environment is critical for any modern mobility ecosystem to thrive, with different players coopeting in some sort of fragile equilibrium but quite often also struggling to gain any competitive advantage, Factual coordinates Molière –short for MObiLIty sERvices Enhanced by Galileo & Blockchain– a Horizon 2020 project co-funded by the EUSPA. Molière has the bold mission of building the world’s best open data commons for mobility services, the so-called “Wikipedia of public transport and new mobility data”, a Mobility Data Marketplace (MDM) developed by Iomob underpinned by blockchain technology, where decentralisation will provide digital trust, transparency, and automatisms in the way mobility data is stored, processed, and used for good. The project will also raise the profile, visibility, availability, and utility of geolocation data (of end users and the vehicles they use) provided by Galileo, among other satellite constellations. In its piloting phase in 2022, Molière will demonstrate how the concepts developed in the project can be applied to high added value use cases, such as to incentivise travellers to use micromobility as a convenient transportation option for trips starting in “transit deserts”, and upgrading an electric car-sharing fleet with state-of-the-art Galileo-enabled On-Board Units to enable more efficient fleet management.
Data is of utmost importance to sustaining effective MaaS that maximises public benefit and increases public transport ridership while establishing a level playing field for privately held mobility services to thrive. Analogous to the widely referenced four levels of MaaS integration topology, four levels of Mobility Management are presented, highlighting the key contribution of mobility data in each level (Figure 3).
Figure 3. Levels of MaaS Integration linked to Levels of Data-led mobility management
Source: Populus
In recent years, many cities have implemented different sorts of Level 1 data-led mobility management by mandating access to mobility datasets from private mobility operators, essentially to oversee their operation in the public right of way. Typically, data is required as a pre-requisite to be granted a license to operate, and such information is basically used to monitor very elementary adherence to city ordinances. SMOU is a remarkable example how a city-led mobility app has incorporated all the information on the available offer of bike-, moped-, and car-sharing in the city of Barcelona.
It is achieved when cities can leverage the data they receive from mobility operators to make more informed decisions and set more effective mobility policies, contributing to alleviating transport externalities, such as congestion and climate impacts, and addressing equity goals.
Examples of data-driven policies that might be implemented by cities to manage mobility fleets include:
- Parking: definition of geospatial areas (geofencing) for preferred and/or restricted parking to protect pedestrian safety or other needs.
- Trips: definition of routes or lanes where micromobility or other types of vehicles have priority over other road users.
- Equity: cities might require or subsidise (see Level 3) fleet services in an area underserved by other means of transport or where specific micro-incentives are put in place where, for instance, first/last mile trips using micromobility services are subsidised only if these trips connect users to public transport hubs (here, continuity of data under a MaaS scheme is needed, so that the policy maker can verify that incentivised trips actually feed public transport, for instance).
- Fleet size: cities might want to set a minimum level of service or maximum fleet size per allowed service area.
A practical example for Level 2 of data-led mobility management, which also includes some aspects of the just mentioned data-driven policies, is the pioneering ">RideSafeUM project. In this EIT Urban Mobility co-funded project, Factual cooperates with micromobility industry leader Dott and other stakeholders to develop an advanced safety solution for micromobility vehicles. The increasing use of micromobility has led to safety concerns for both drivers and pedestrians. Within the project, a generic and versatile solution is being developed, enabling data-driven decision making. Thus, data from mobility operators can be used to define more effective mobility policies.
Factual also takes part in the Horizon 2020 project nuMIDAS, where new and emerging mobility trends are analysed with a view to reviewing and assessing a range of options for collecting and using new mobility data by means of new data collection and management approaches, including new methods and tools to exploit said data while encompassing Artificial Intelligence / Machine Learning techniques for dealing with Big Data.
Given that most travel decisions are largely influenced by time and cost, pricing is an incredibly important tool for public agencies to leverage in shaping desired transportation outcomes.
Mobility management is achieved when cities effectively leverage pricing strategies, including subsidies, to influence how travellers decide whether to walk, drive, use public transport or shared mobility services, or hail a ride.
Many cities overseeing shared micromobility programmes have begun to implement more complex policies for pricing the public right of way, including parking fees (applied to restricted parking areas), fines for non-compliance with equity policies, and fees for riding in unauthorised zones.
While setting a fair price for access to roads, kerbs, and on/off-street parking is an important mechanism for cities to influence traveller behaviour, subsidies are also important. Public transportation services are typically heavily subsidised because they are viewed as providing a significant public utility –in short, public transport enables cities to move more people more efficiently than would otherwise be possible if they each drove individual vehicles.
Similarly, as new private mobility services continue to expand, it is important for cities to determine when and where private mobility services provide social benefit in order to consider subsidising them.
It goes without saying that public subsidies must be implemented in the most transparent, cost-efficient, and targeted way, and be designed to address Key Performance Indicators included in cities’ mobility plans.
Related to the above, Rideal is a versatile Software as a Service (SaaS) platform that Factual’s Venture Builder, Keita Mobility Factory, has developed and that can be plugged in to any existing MaaS or mobility operator backend platform and be used to design and manage micro-incentive programmes to nudge behavioural change towards more sustainable mobility and monitor its effectiveness in real time.
Subsidising shared mobility with public money is not new. For example, prior to the emergence of venture-backed electric scooter companies, the majority of bikeshare systems received public subsidies. Moreover, important industry stakeholders, such as the UITP or POLIS, to name but a few, are embracing the idea of micro-incentives to any mobility service available in a city as a way to implement more efficient mobility strategies, where the availability of data provided by / shared through MaaS platforms is critical.
Key examples of when cities may wish to provide incentives to private mobility operators include:
- Supporting first/last-mile access to mass transit, for instance by incentivising micromobility services.
- Expanding transportation access in underserved areas, for instance by incentivising ride-hailing or DRT.
- Shifting travellers to certain modes, and in certain time frames, to reduce congestion during peak transit times, flattening demand curves.
- Supporting (incentivising) mobility services that have a lower carbon footprint than personal vehicles.
Today, many cities have realised Level 1, 2, and perhaps 3 of mobility management, though micro-incentives are still a nascent idea which Factual is proposing through Rideal.
Third-party mobility data analytics platforms deliver Levels 1 to 3 mobility management solutions to enable cities to manage multiple shared mobility services, including shared bikes, scooters, mopeds, and cars, but few cities have implemented mobility management beyond micromobility, and cities are struggling to effectively implement mobility management policies to optimise across the multiple transportation modes other than micromobility.
Public agencies will be able to influence how travellers make transportation decisions across modes to promote societal goals: reducing transportation climate impact, alleviating congestion, and expanding equitable access to mobility. To reach Level 4 mobility management, cities will need access to data from the various transportation services delivered on their public right of way to make data-driven decisions, including the implementation of pricing and incentives. Level 4 mobility management can more easily be achieved alongside Level 4 MaaS solutions. That is, the mechanism through which real-time information about transportation options, specifically new pricing and subsidies, could be more easily delivered to a large population of travellers through one, or more likely multiple, MaaS consumer-facing applications.
Mobility is a key aspect of our society. New mobility services and innovations are appearing in the mobility landscape, but there is still room for improvement with regards to data management and its associated added value. From the public perspective, cities will need information from data exploitation for better mobility planning in order to achieve effective, inclusive and sustainable mobility by setting a clear and fair data-sharing framework with mobility operators that identifies the information needed and the processes for its collection, sharing and storing, among other aspects, such as privacy and GDPR compliance. In order to achieve this objective, a minimum level of trust between public and private partners is needed, where we describe three approaches to mobility data sharing: aggregated data by mobility operators –such as most carsharing operators–, data consolidated by a trusted third party –this can be an academic institution, not-for-profit or data analytics platform–, or cities requesting raw and disaggregate data –as with the MDS and CDS-M initiatives. The decision should consider the price tag for data and the acceptable level of risk, and it must be selected on a case-by-case basis. Data sharing is also a key driver for progressing in the four levels of MaaS integration, with the current status of many cities being Level 1, which refers to integrated information and mobility data reporting but where the expected added value that data-led mobility management can reach is the promotion of societal goals and multimodal optimisation through innovative tools such as micro-incentives platforms. Hence, the addition of a data management layer to the new mobility initiatives means that cities will be closer to reaching the objectives of more effective, inclusive, and sustainable mobility.
KOROSEC, Kirsten (2021). “Via buys mapping startup Remix for $100 million”. In: Tech Crunch+ [online]. Available at: https://techcrunch.com/2021/03/09/via-buys-mapping-startup-remix-for-100-million/?guccounter=2. [Accessed: February 2022].
Open Mobility Foundation (OMF). “About MDS”. Open Mobility Foundation [online]. Available at: https://www.openmobilityfoundation.org/about-mds/. [Accessed: February 2022].
SOCHOR, Jana; ARBY, Hans; KARLSSON, MariAnne; SARASINI, Steven (2018). “A topological approach to Mobility as a Service: A proposed tool for understanding requirements and effects, and for aiding the integration of societal goals”. Research in Transportation Business & Management, vol. 27, pàg. 3-14. DOI: https://doi.org/10.1016/j.rtbm.2018.12.003.
TEALE, Chris (2020). “Uber sues LADOT over data-sharing requirements”. Smart Cities Dive [online]. Available at: https://www.smartcitiesdive.com/news/uber-jump-sues-los-angeles-mobility-data-sharing-requirement/574893/. [Accessed: February 2022].
TOMP Working Group (TOMP-WG). “About the TOMP-API”. TOMP Working Group [online]. Available at: https://tomp-wg.org/. [Accessed: February 2022].
LABORDA, Josep<. Mobility data management and its potential to generate value. Oikonomics [online]. May 2022, n. 18. ISSN: 2339-9546. DOI: https://doi.org/10.7238/o.n18.2217
ODS
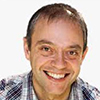
Co-founder and CEO of FACTUAL, an innovation consultancy focused on mobility. MsC in Telecommunications for the Polytechnical University of Catalonia (UPC). He has participated in more than 20 European research and innovation projects, some of them as a coordinator, targeting more sustainable, intelligent and connected mobility. He also advises public administrations at an international level for the effective deployment of MaaS services in terms of governance and data sharing. Former member of Strategy Committee at ERTICO and MaaS Alliance, he contributes as an expert reviewer for the European Commission. He is an associate consultant for Cities Forum on MaaS and Smart mobility